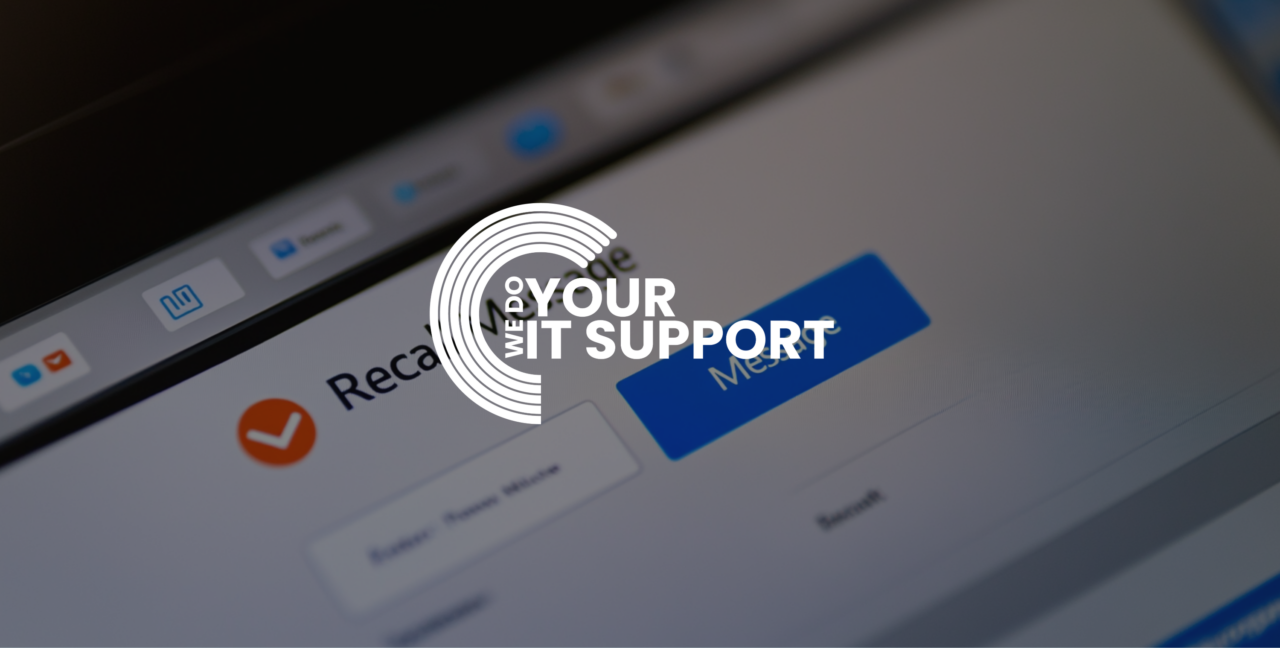
The allure of Google Gemini AI lies in its proclaimed advanced capabilities and innovative features, including sophisticated natural language understanding, multimodal abilities, scalability, adaptability, impressive speed, and accuracy. Google AI Studio emphasises that their models undergo extensive ethics reviews. However, recent evaluations from esteemed institutions like Cornell University and the University of California, Santa Barbara, reveal disparities between these glowing assertions and the model’s real-world performance. Specifically, Google Gemini falls short in global reasoning tasks and struggles with analysing large-scale datasets, posing notable challenges for businesses in sectors like finance and healthcare that rely heavily on precise data interpretation. Additionally, when it comes to video analysis or recognising objects in images, Gemini’s performance leaves much to be desired. While Google maintains a strong promotional stance, these findings underscore the importance of bridging the gap between marketing claims and operational realities. The use of an extended context window and the development of a truly capable model will be crucial in meeting the practical needs of users and maintaining transparency about the model’s limitations.
Despite the buzz surrounding Google’s Gemini AI models, recent findings suggest a discrepancy between their marketed potential and actual performance. Institutions such as Cornell University and the University of California, Santa Barbara, have highlighted deficiencies in Gemini’s capabilities, particularly with global reasoning tasks and the analysis of large-scale datasets. These shortcomings become critical for businesses in sectors like finance and healthcare, where precise data interpretation is crucial. Additionally, the models demonstrate inconsistent performance in video analysis and object recognition in images. Even with the introduction of the Gemini API and advancements in Google Cloud Vertex AI, these issues persist across different versions, including Gemini 1.5 Pro and Gemini Nano. While Google DeepMind continues to promote the advancements and continual improvements of these large-scale foundation models, these insights stress the necessity for ongoing R&D to bridge the gap between ambitious claims and operational functionality. Ensuring users can rely on the models’ practical utility remains a key challenge.
The central claim that Google’s large scale foundation model, Gemini 1.5 Flash, can handle large datasets with speed and accuracy has come under scrutiny. Recent studies from Cornell University and the University of California, Santa Barbara, indicate that Gemini Ultra’s highly touted capabilities are not fully realised in practice. When tasked with analysing extensive datasets, these capable AI models frequently encounter difficulties, particularly in global reasoning tasks and speculative fiction with complex world-building. This has significant implications for industries such as finance and healthcare, where the precision of data analysis is paramount. While Google’s promotional materials emphasise advances in generative AI scalability and adaptability, the discrepancies highlighted by these studies suggest a need for enhanced R&D to align Gemini Advanced’s real-world performance with its marketed potential. As enterprise customers increasingly depend on AI for critical decision-making, the importance of transparent and reliable AI capabilities cannot be overstated.
The performance of Google’s Gemini models has come under intense scrutiny, with recent studies from prestigious institutions such as Cornell University and the University of California, Santa Barbara, revealing significant gaps between the models’ marketed capabilities and their actual performance. These AI models, including the Gemini Ultra and Gemini Advanced, are praised for their advanced natural language understanding, multimodal abilities, longest context window, and impressive speed and accuracy. However, they have shown to falter notably in tasks involving global reasoning and the analysis of large-scale datasets. Such shortcomings pose considerable challenges for industries like finance and healthcare, which depend on precise data interpretation for decision-making. Additionally, the models’ inconsistent performance in video analysis and object recognition highlights the necessity for Google to intensify R&D efforts, ensuring that practical utility and transparency are maintained. While Google continues to spotlight the evolutions and improvements of the Gemini models, including the Gemini 1.5 Pro, these findings underscore the critical need for bridging the gap between ambitious promotional claims and actual operational functionalities. Furthermore, the accessibility of these models via an API key is crucial for developers seeking to integrate them into their applications.
The recent evaluations of Google’s Gemini models by institutions like Cornell University and the University of California, Santa Barbara, highlight significant discrepancies between the AI’s marketed potential and its actual performance, particularly in crucial areas such as global reasoning and large-scale data analysis. These inaccuracies can have profound implications for businesses, especially those in sectors like finance and healthcare that rely on precise data interpretation for critical decision-making. Missteps in AI interpretations could lead to faulty conclusions, affecting everything from financial forecasting to patient diagnostics. Furthermore, the models’ inconsistent capabilities in video analysis and object recognition underscore the need for businesses to remain cautious and well-informed about the tools they integrate into their operations. With the integration of Vertex AI, Gemini Nano models, Google Maps, and Duet AI, businesses must ensure that these neural networks and generative AI solutions are thoroughly vetted. The Gemini API and Google DeepMind’s involvement make it even more critical for companies to engage in rigorous R&D verification to ensure that AI solutions like Gemini can deliver on their promises. Transparent and reliable AI is not just a technological need but a business imperative.
Recent research conducted by Cornell University and the University of California, Santa Barbara, has raised substantial concerns regarding the real-world performance of Google’s Gemini AI models, including the Gemini Pro and Gemini 1.5 Pro. Despite Google’s claims of impressive capabilities in natural language understanding, multimodal processing, and data analysis, the studies found notable discrepancies. Specifically, the Gemini models struggled with global reasoning tasks and the analysis of large-scale datasets—both critical functions for industries such as finance and healthcare that depend on accurate data interpretation. Additionally, the models exhibited inconsistent performance in video analysis and object recognition tasks, further questioning their reliability and practical utility. These findings underscore the necessity for more rigorous R&D from Google DeepMind to align the models’ operational results with their marketed promises and highlight the importance of transparency and reliability in AI technologies like Vertex AI for business decision-making. Ensuring efficient models through comprehensive safety tests will be crucial moving forward.
The recent findings that uncover notable shortcomings in Google’s Gemini models present a unique opportunity for competitors in the AI landscape. As Google’s AI Studio struggles with global reasoning, large-scale data analysis, and exhibits inconsistent performance in critical areas like video analysis and object recognition, rivals can capitalize on these gaps. With Gemini models showing particular weaknesses in neural network efficiency and mathematical reasoning, competing AI developers can focus their R&D efforts on overcoming these precise limitations. Moreover, by enhancing on-device tasks and optimising for mobile devices, they can position their solutions as more reliable and effective alternatives for industries demanding precision and accuracy, such as finance and healthcare. This situation underscores the competitive nature of the AI market, where technological advancements and setbacks can rapidly shift industry dynamics, offering a window for competitors to gain a foothold and potentially erode the dominance of Google products in the AI domain.
Given the keen scrutiny and subsequent findings by institutions such as Cornell University and the University of California, Santa Barbara, the crucial question arises: Can Google bridge the gap between the theoretical capabilities and practical utility of their Vertex AI models, including Gemini 1.5 Pro, Gemini 1.5 Flash, and Gemini Ultra? Addressing the identified weaknesses in global reasoning, large-scale data analysis, video analysis, and object recognition is vital. As these areas are critical for sectors like finance and healthcare, Google’s ability to enhance the practical utility of their Vertex AI and generative AI models will significantly impact their market positioning. To achieve this, increased investments in research and development are imperative, aiming to rectify performance inconsistencies and align the models’ real-world functionalities with their ambitious claims. Success in this endeavor, particularly with efficient models like Duet AI, will not only reaffirm Google’s leadership in the AI landscape but also restore businesses’ confidence in deploying Gemini models for critical decision-making processes.
As AI continues to advance, the development of more reliable and transparent models becomes paramount, especially for businesses operating in data-intensive sectors like finance and healthcare. While Google’s Gemini AI models have faced scrutiny due to their performance inconsistencies, these challenges highlight the broader landscape of generative AI model evolution. Future advancements will likely centre on improving data interpretation accuracy, global reasoning capabilities, and enhancing functionalities in complex tasks such as video analysis and object recognition. This ongoing progress in generative AI is not just a technological pursuit but a business necessity, offering companies the dual benefits of innovation and risk mitigation. For businesses, staying attuned to these developments means not only adopting cutting-edge generative AI solutions but also engaging in thorough vetting processes and contributing to the research landscape to ensure that the AI tools they deploy are both effective and trustworthy.