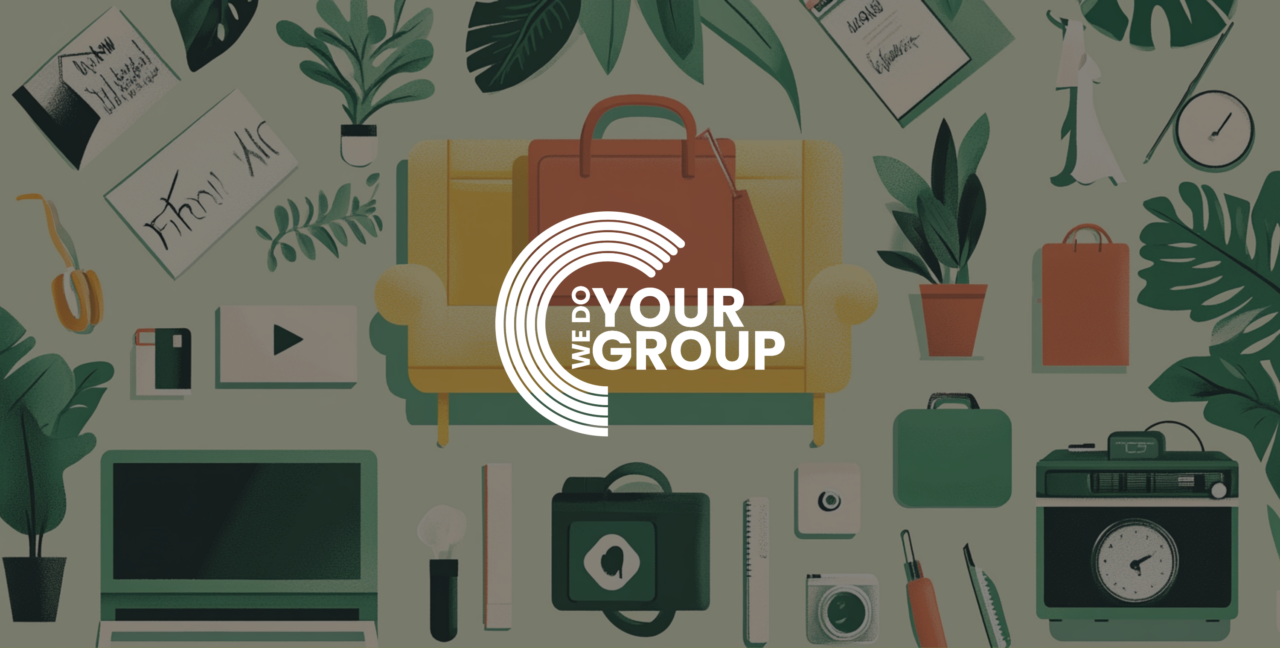
In this insight, we look at what ‘data gravity is, what challenges it creates, and how businesses can tackle data gravity challenges.
Working with (larger) datasets means collecting, storing and managing the data and moving it around to different applications. The data then accumulates (builds mass) and attracts services and applications which need to be close to the data to improve the latency and throughput and high leverage bandwidth. As more data collects and grows at a specific location / a central data store (on-premises or co-located), the process accelerates to the point where it’s difficult or impossible to move data and applications anywhere else. This affects workflows, creates higher costs, and results in lower system performance and management overheads.
The term for this cumbersome, dragging effect of a central store data of costly, challenging to manage data on a business was, therefore, first dubbed ‘data gravity’ by IT researcher Dave McCrory in 2010.
So-called ‘artificial’ data gravity can also occur when attractive forces are created through indirect or outside influence, such as costs, throttling, specialisation, legislation, or usage.
For example:
– With cloud storage, although the cloud allows fast scalability, large and growing datasets stored there also attract analytics and applications, and more cloud storage egress fees (charged when applications write data out to the network or repatriate data back to the on-premises environment).
– Usage, e.g. Dropbox charges each user for the use of Shared Data (Artificial Usage), so each person pays for the data consuming their storage, but Dropbox only stores and directs authorised users to a single copy.
Therefore, artificial data gravity is a product of cloud services’ financial models, not technology.
Ways in which businesses and organisations can try to tackle data gravity challenges include:
– Separating data storage by utilising event-driven architectures.
– Investing in new storage solutions, e.g. solid-state storage or tiering and storage management tools.
– Using hyper-converged systems, i.e. consolidating resources and reducing costs by combining computing, storage, networking, and management in one unified system. This, however, can have scalability challenges.
– Using cloud-based solutions. This can require using Cloud Architects (cloud management specialists), Cloud-native applications such as Amazon QuickSight, or cloud gateways and cloud-native technologies (container-based environments), e.g., object storage.
– Opting for a multi-cloud strategy (to reduce vendor dependency), using cloud-native storage tiers, e.g. on AWS, Google Cloud, and Azure and matching them to the performance and access frequency of different types of data processing.
– Scaling public cloud computing for batch processes and large-scale analysis.
– Closely monitoring costs to ensure there are no data gravity cost hotspots.
– Making greater use of analytics (analysing data at the edge) and developing better data management and governance strategies.
For businesses that collect large amounts of datasets, managing that data in a cost-effective way and in a way that maintains workflow is a severe issue. Keeping a close eye on costs and analytics, making better, smarter use of the cloud, taking specialist cloud advice, and using cloud-native applications are some ways businesses can avoid falling victim to the effects of costly and cumbersome data gravity. Although a proportion of the data collected may generate value for businesses, too much data in one location can reduce that value by attracting costs and creating an issue that can affect competitiveness. Recognising and understanding what data gravity is and how it occurs, coupled with more of a focus on data management and planning, can prevent data gravity problems in the future.